Leveraging trip data to gain actionable insights into urban mobility in Chicago
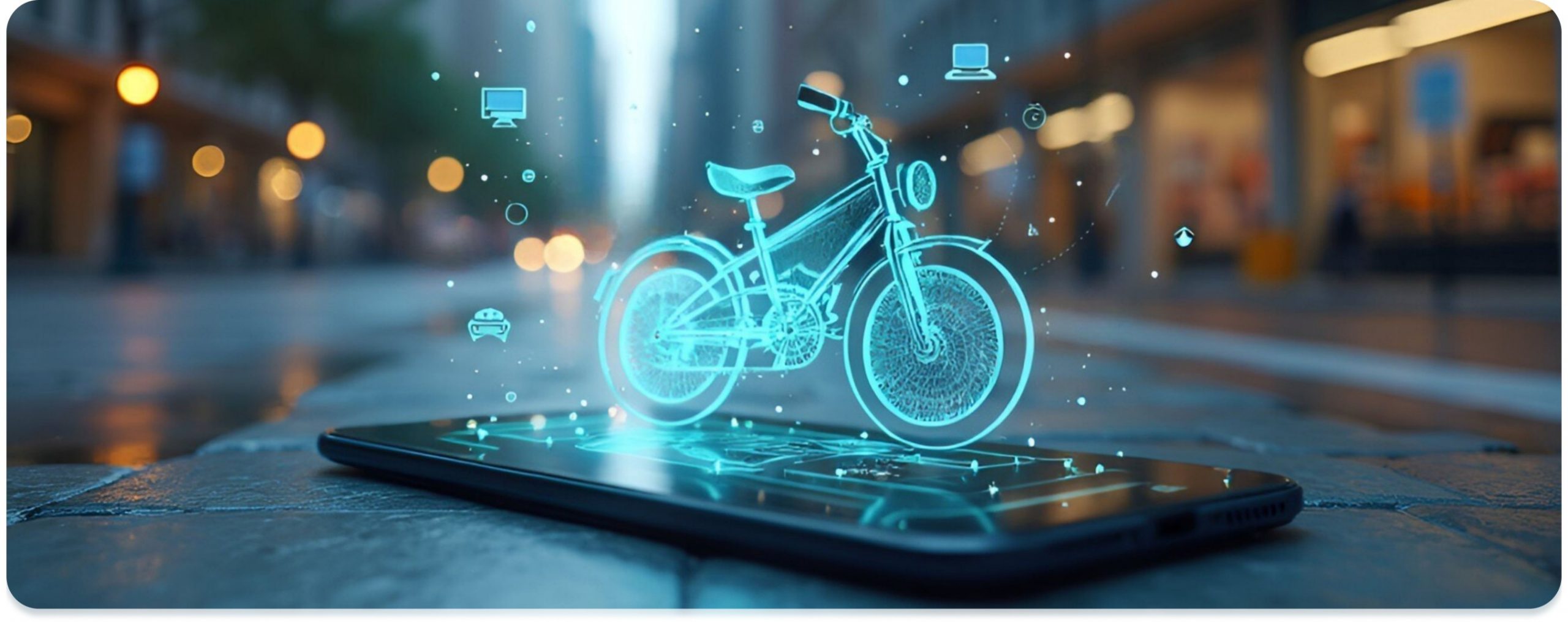
Introduction
Cyclistic Bike-Share is a transformative bike-sharing program in Chicago that promotes sustainable urban transportation. This study utilizes historical trip data to analyze user behaviors, geographic patterns, and temporal trends, aiming to enhance service delivery and urban planning.
The dataset contains extensive information about bike rides, such as trip duration, bike type, start and end locations, and user classifications. This data allows for a detailed exploration of how different factors impact bike-share usage and provides actionable insights into improving transportation services.
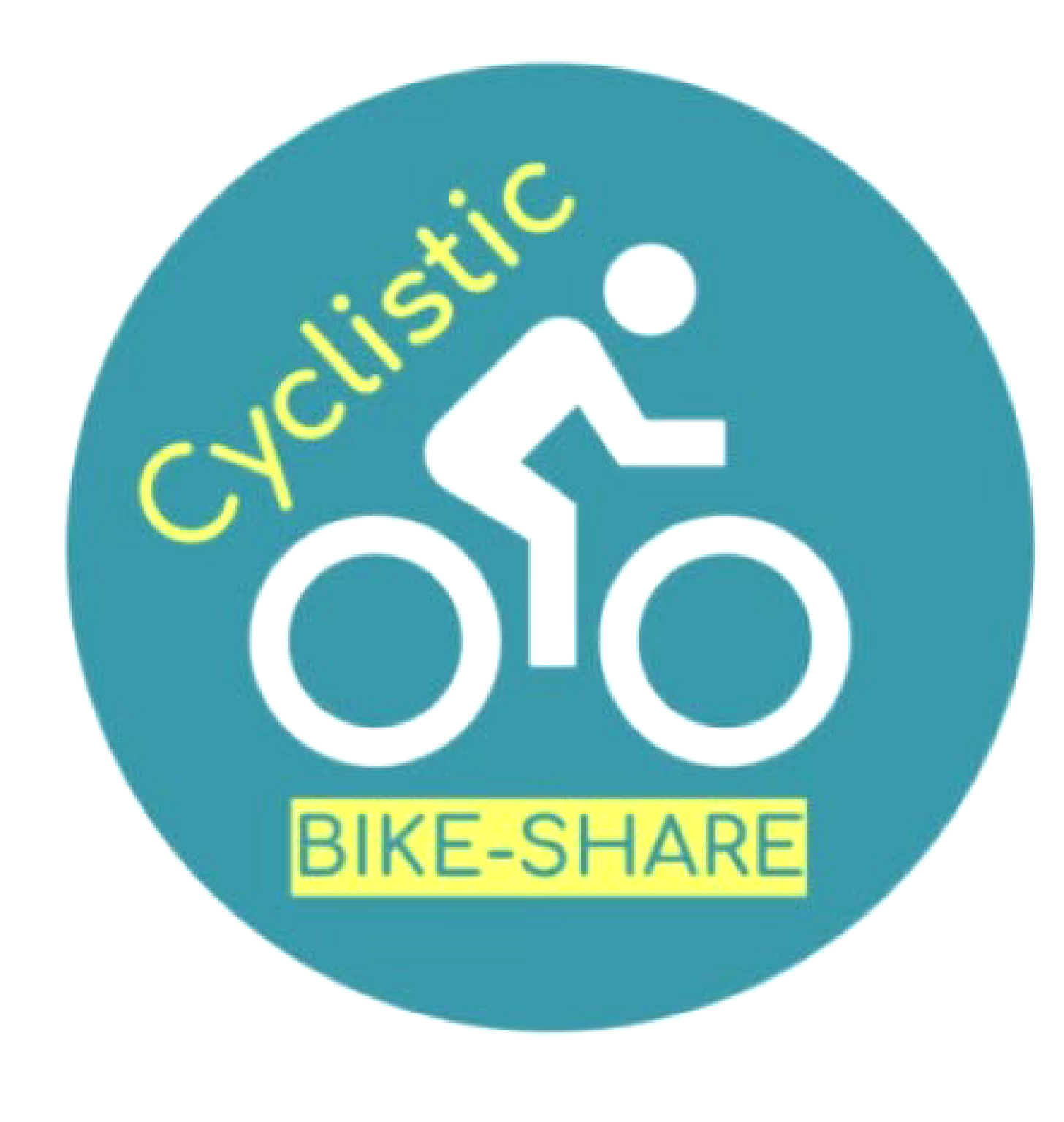
Objective
To extract meaningful insights from the Cyclistic Bike-Share Dataset, this analysis focuses on:
- Identifying temporal patterns in ride behavior.
- Understanding usage differences between casual riders and members.
- Exploring geographic trends to optimize service locations.
- Gaining insights into ride durations based on time and demographic factors.
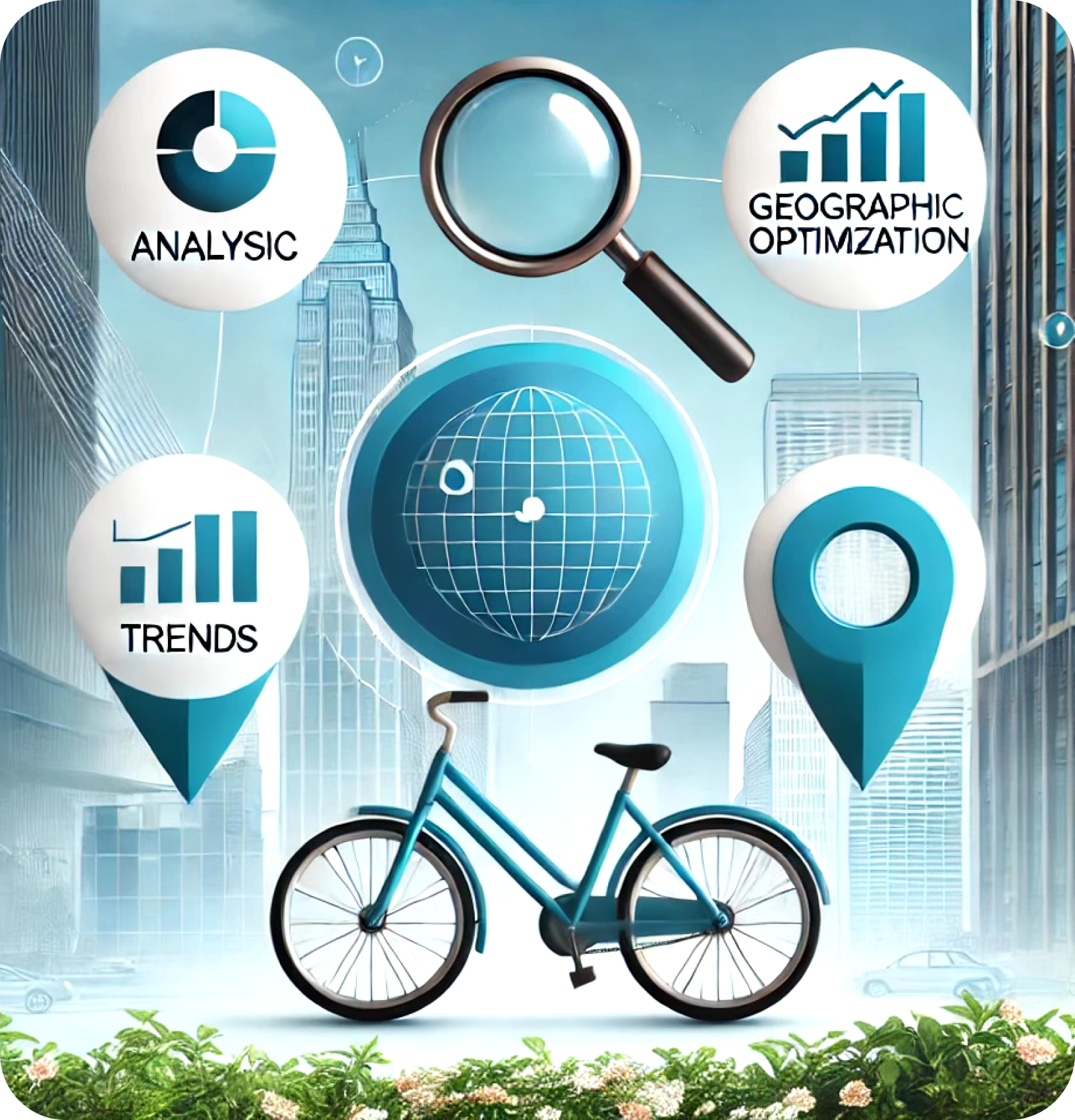
Methodology
-
1. Data Preparation:
- Combined monthly and quarterly datasets from 2018 to 2022.
- Engineered features like ride duration, day of the week, hour of the day, and duration bin.
-
2. Analysis Tools:
- Used Plotly and JavaScript to create interactive visualizations.
- Incorporated dropdowns and sliders for data filtering and customization.
-
3. Visualization Framework:
- Designed a set of interactive plots to reveal key patterns in the data.
Visualizations
Heatmap: Start Locations of Bike Rides
- Description: A geospatial heatmap visualizing ride density across Chicago neighborhoods. Filters allow exploration by user type, bike type, and day of the week.
- Insight: Popular starting points highlight high-demand locations, aiding resource allocation.
- Significance: Understanding spatial usage patterns helps optimize bike availability and service planning.
Average Ride Duration Over Time
- Description: A time-series line graph illustrating changes in average ride duration over the dataset period, filtered by user type.
- Insight: Peaks and troughs reflect seasonal variations and major events impacting ride durations.
- Significance: Helps in forecasting demand and adapting service strategies.
Ride Duration by Hour of Day
- Description: A bar graph showing how ride durations vary across hours, with filters for duration bins and user types.
- Insight: Highlights peak hours for rides, typically aligning with commuter patterns.
- Significance: Guides operational decisions such as bike redistribution and maintenance schedules.
Average Ride Duration by Day of Week
- Description: A bar chart comparing average ride duration for each weekday, segmented by duration bins and user types.
- Insight: Identifies differences in ride behavior between weekdays and weekends.
- Significance: Enables targeted promotions and better service alignment with user needs.
Average Ride Duration by Hour of Day and Day of Week
- Description: A bar chart comparing average ride duration for each weekday, segmented by duration bins and user types.
- Insight: Identifies differences in ride behavior between weekdays and weekends.
- Significance: Enables targeted promotions and better service alignment with user needs.
Average Ride Duration by Hour for Selected User Type
- Description: A line graph allowing users to explore average ride duration by hour for members or casual users. An interactive slider facilitates hour-by-hour analysis.
- Insight: Provides an understanding of when different user groups are most active.
- Significance: Helps in designing tailored services for specific user groups.
Average Ride Duration by Hour for Selected User Type
- Description: A funnel plot showing average ride duration for each weekday, with percentage comparisons.
- Insight: Highlights weekdays with the longest or shortest rides, informing targeted strategies.
- Significance: Useful for marketing and understanding weekly ride patterns.
Summary
This analysis of Cyclistic’s bike-share data reveals crucial insights into urban mobility:
- Temporal Trends: Commuting patterns dominate weekday rides, while weekends attract more casual riders.
- User Behaviors: Members tend to have consistent, shorter rides, whereas casual users show variability in duration.
- Geographic Insights: Key hotspots for bike-sharing services indicate areas requiring increased resources.
By leveraging these findings, Cyclistic can refine its services, improve user satisfaction, and support Chicago’s goals for sustainable urban transportation.
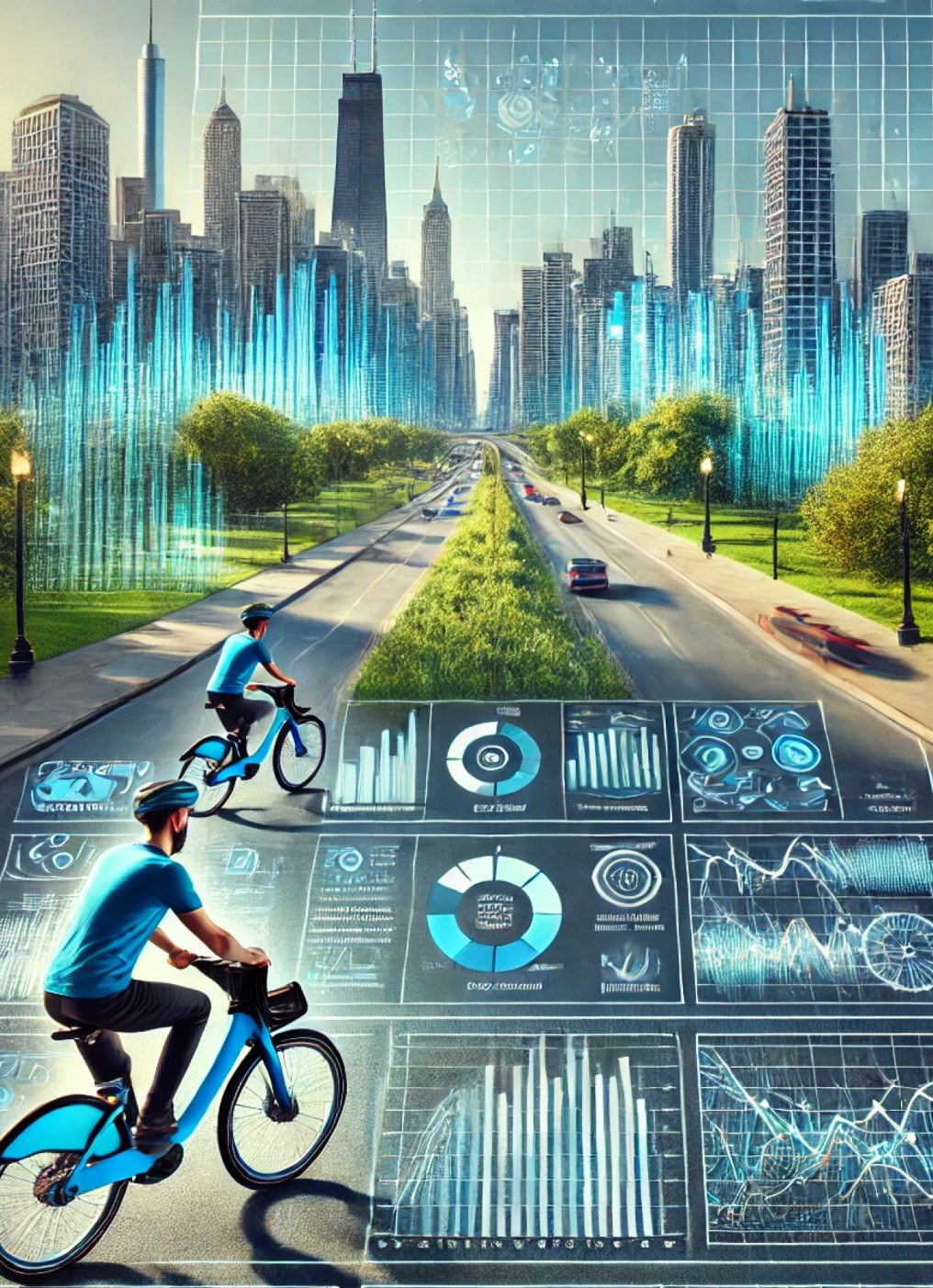