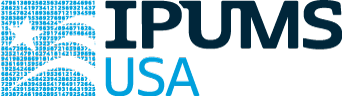
Introduction
Income inequality is a pervasive issue that has far-reaching economic, social, and political implications. Understanding the factors that contribute to disparities in income is critical for developing policies and strategies to promote economic equality. This project focuses on conducting an in-depth Exploratory Data Analysis (EDA) of income-related variables within the IPUMS USA dataset, which provides a comprehensive and representative sample of demographic and socioeconomic data from the U.S. population.
The primary objective of this project is to explore and analyze how various demographic factors—such as age, gender, education, and occupation—affect income distribution. Through this analysis, we aim to uncover patterns of income inequality and identify potential drivers that contribute to income disparities. The dataset’s rich set of variables offers an opportunity to examine not only the general distribution of income across the population but also the nuances of how different socioeconomic characteristics influence earning potential.
Objective
- Conduct EDA on income variables to understand income distribution and inequality.
- Investigate the relationship between demographic factors (e.g., age, gender, education, occupation) and income.
- Measure income inequality and explore its variation across different demographic groups.
- Provide insights and recommendations based on the analysis of income disparities.
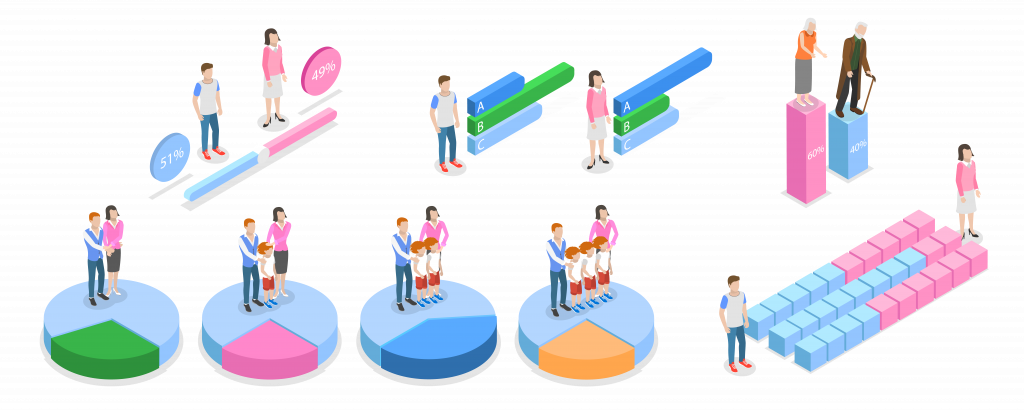
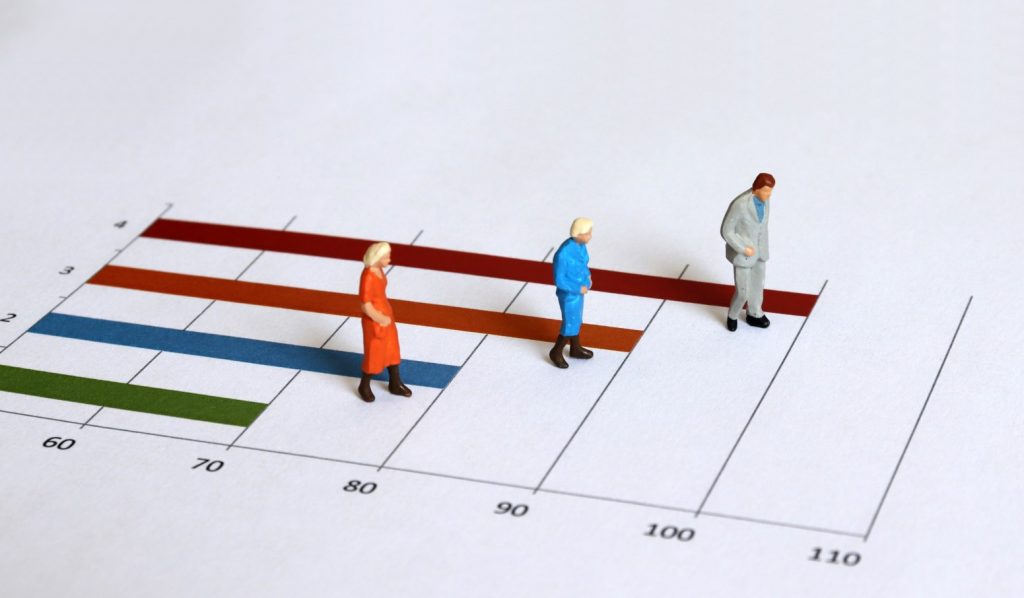
Methodology
Data Loading and Cleaning
- The IPUMS USA dataset was loaded into a Pandas DataFrame using an API key.
- The dataset was checked for missing values, and no missing values were found.
Variable Selection
- Relevant variables, including income, age, gender, education level, and occupation, were selected for analysis.
- Outliers were removed, and income-related variables were standardized for further analysis.
Descriptive Statistics
- Summary statistics for income were calculated across demographic groups (e.g., age, gender, education) to explore income distribution.
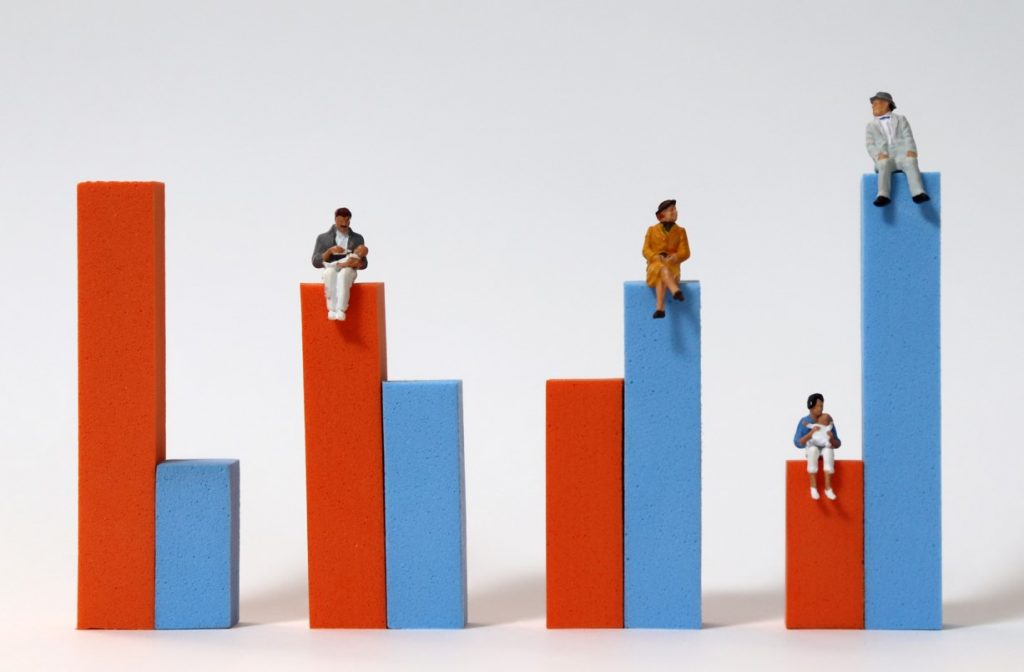
Visualization
- Histograms, box plots, and heat maps were created to visualize income distribution and inequality across different demographic categories.
Analysis
- T-tests and ANOVA were performed to assess the impact of demographic and socioeconomic factors on income.
- The Gini coefficient was calculated to measure income inequality and analyze variation across demographic factors like gender, race, and age.
- The relationships between education level, occupation type, and income were explored through correlation analysis.
Results
Education, Employment, and Race Insights
Income and Education Trends
Income Brackets and Employment
Summary
This exploratory data analysis on income variables revealed significant disparities in income distribution, influenced by factors such as education, gender, and occupation. The analysis also provided a clear picture of income inequality, with the Gini coefficient illustrating the extent of the disparities. These findings offer valuable insights for policymakers, educators, and organizations seeking to address income inequality and promote more equitable economic opportunities.
The results emphasize the importance of reducing gender-based income disparities, increasing access to education, and providing more equal career advancement opportunities. Ultimately, the project demonstrates that while education and occupation remain central to income outcomes, systemic factors such as gender and age must also be addressed to reduce overall income inequality in society.
Team Members
Name | Role |
---|---|
Hasaranga Dilshan Jayathilake | Lead Data Analyst |
Supraja Pericherla | Lead Data Analyst |
Junaid Ahmed Mohammed | Data Analyst |
Bhavya Karla | Data Analyst |
Abhiram Yalavarthy | Data Analyst |
Saundarya Pande | UI/UX Designer |
Narasimha Rohit Katta | Web Developer |
Navya Kamepalli | Project Manager |