Revealing Educational Trends: Outlier Analysis of SAVI Data for School Corporations (25) and Counties (12) Using R.
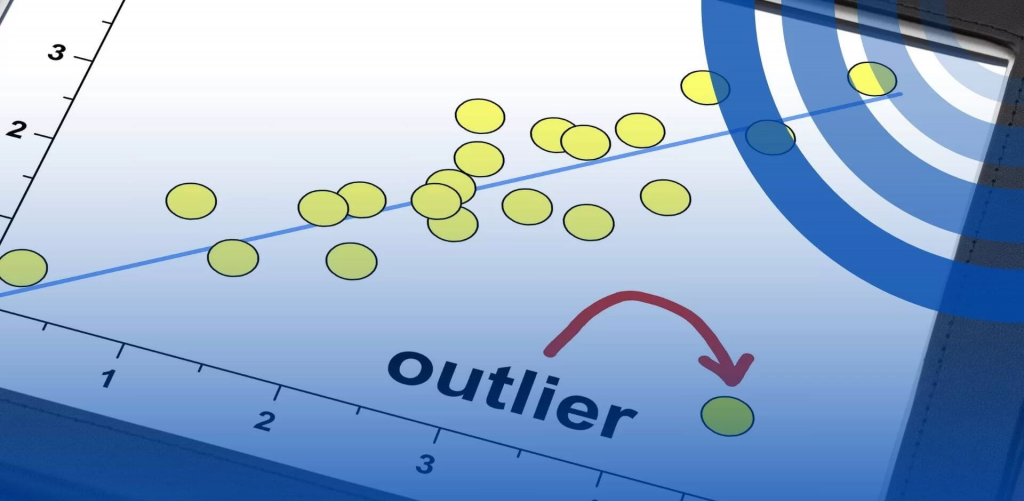
Introduction
Our project aims to detect outliers in a dataset containing educational health indicators for school corporations and counties over multiple years for SAVI and Evansville datasets. Using robust statistical techniques, we seek to uncover insights into public health trends and educational impacts. The dataset includes records with year, geography, and indicator information, enabling us to conduct detailed outlier analysis for both school corporations and counties.
We also use geographic contextual data, providing unique identifiers and descriptive details for each location, to map data points accurately. This analysis, along with indicator visualization data, will help inform educational policies and public health strategies, ultimately contributing to improved educational outcomes and public health initiatives.
Methodology
1. Data Cleaning:
– The dataset containing information about geographies, data values, years, indicator IDs, and display labels was cleaned to remove missing or erroneous data points, ensuring the reliability of our analysis.
– We standardized the data format and checked for any inconsistencies or anomalies in the data values.
2. Data Analysis:
– Mean and standard deviation were calculated for the indicators across school corporations and counties, providing insights into central tendencies and variabilities.
– Bias and trend calculations were performed for each indicator over the years, revealing significant trends or biases in the data.
3. Data Visualization:
– Using Tableau Public, we created user-friendly plots to effectively communicate our findings.
– Plots were generated to showcase outlier trends and percentages of counties and school corporations for each indicator and geography.
– These visualizations facilitated a clearer interpretation of the data and enabled us to communicate our results to stakeholders effectively.
Workflow

Visualizations
Tree Map Visualization:
Visualized the distribution of outlier counts across different indicators and geographies (counties and school corporations). The treemap highlighted significant regional disparities in outlier occurrences. Greater Jasper and Vanderburgh School Corporations emerged as hotspots, as having the highest number of outliers (51 and 50each).
Pie Chart Visualization:
Showcased the percentage of outliers for each indicator within the SAVI and Evansville datasets. And also incorporated filters for trend and geography to facilitate comparative analysis. By filtering by geography, we could compare the distribution of outliers across counties and school corporations. This provided insights into regional differences in data quality and potential sources of variation.
Bar Chart Visualization:
The bar chart clearly illustrated the concentration of outliers in certain counties and school corporations. This information can help prioritize investigations and resource allocation. By analyzing the relationship between trend and outlier count, we can identify indicators that exhibit significant deviations from expected patterns.
Summary
- Conducted dataset analysis, selecting pertinent columns for further examination to ensure relevance and accuracy.
- Calculated statistical measures such as mean, trend, bias, and standard deviation to gain insights into the dataset’s central tendencies and variabilities.
- Identified outlier counts for each indicator and geography in both counties and school corporations, highlighting significant deviations from expected values.
- Utilized visualization techniques to present outlier trends and indicator distributions, facilitating a deeper understanding of the dataset and its implications.
Team Members
Name | Role |
---|---|
Hasaranga Jayathilake | Lead Data Analyst |
Supraja Pericherla | Lead Data Analyst |
Sri Naga Venkata Vijay Kumar Pulavarthi | Data Analyst |
Sai Aravind Donga | Data Analyst |
Rakesh Reddy Yennam | Data Analyst |
Padmavathy Kandasamy | UI/UX Designer |
Rutika Chandrashekhar Bangera | Web Developer |
Aakriti Bhandari | Project Manager |